- Ph.D., City University of New York, 2001
- Sci.M., Nankai Institute of Mathematics, 1995
- B.Sc., University of Science and Technology of China, 1992
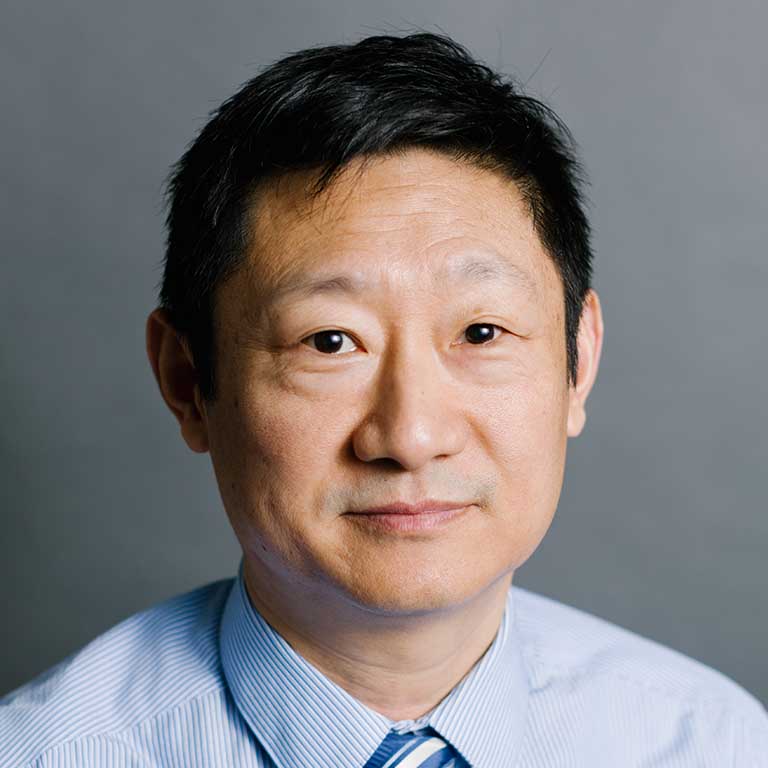
Hu Cheng
Senior Scientist, Psychological and Brain Sciences
Physicist, Imaging Research Facility
Senior Scientist, Psychological and Brain Sciences
Physicist, Imaging Research Facility
As an MRI physicist, my primary responsibility lies in overseeing MRI-related image quality control and protocol optimization for neuroimaging applications. Presently, my research is concentrated on four key areas: functional MRI, diffusion MRI, MR spectroscopy, and deep learning. My overarching goal is to bridge the technical advancements in these domains and translate them into valuable clinical applications.
Distinct from conventional MRI, MR spectroscopy (MRS) presents a unique imaging technique with shared underlying principles. It allows for the quantification of various brain metabolites in vivo, holding significant potential in identifying biomarkers for diverse brain disorders. Diffusion MRI is a unique noninvasive technique that can infer the microstructure information of brain tissues. It encodes the diffusion of water molecules in the tissue by applying external diffusion-sensitized magnetic gradients with different amplitudes and directions. The signal variation of each voxel is determined by the underlying microstructure, which can be decoded by proper post-processing and modeling. We are particularly intrigued by the application of MRS and diffusion MRI to explore neurometabolic and microstructural changes triggered by subconcussive impacts. Specifically, our focus is on advancing diffusion MRS techniques to discern signals originating from glial cells and neurons, thereby enhancing our understanding of these processes.
In the realm of functional MRI, we have made substantial strides in multiple techniques, encompassing network analysis, physiological noise reduction, and sparse acquisition. We are also interested in functional MRI in the brain stem evoked by tougue movement.
As deep learning made its tremendous success in data mining and artificial intelligence, we have dedicated significant effort to mastering this transformative technique. We have proposed a 1D CNN-based deep learning method to reduce sum-of-square noise in diffusion MRI retrospectively. We also emplyed a U-NET based segmentation method to extract the right ventricle of cardiac MRI images. We are committed to further harnessing the potential of deep learning in advancing image processing techniques.
In essence, my research is underpinned by a deep commitment to refining MRI technologies, and my aspiration is to seamlessly integrate these advancements into clinical practice and brain research, ultimately enhancing our understanding of how the brain works in normal and complex neurological conditions.
Cheng, H., Wang Y., Sheng, J., Sporns, O., Kronenberger, W.G., Mathews, V.P., Hummer, T., Saykin, A. (2012). Characteristics and variability of structural network derived from diffusion tensor imaging. Neuroimage, 61:1153-64.
Cheng, H. (2012). Variation of Noise in multi-run fMRI using GRAPPA. Journal of Magnetic Resonance Imaging, 35:462-70.
Cheng, H., Wang Y., Sheng, J., Sporns, O., Kronenberger, W.G., Mathews, V.P., Hummer, T., Saykin, A. (2012). Effect of Number of Seeds in Tractography on the Variance of Structural Networks. Journal of Neuroscience Methods, 203:264-72.
Kim, D., Patrick, D.S., Cheng, H., Pruce, B.J., Braumbaugh, M.S.m Vollmer, J.M., Hetrick, W.P., O'Donnell, B.F., Sporns, O., Puce, A., Newman, S.D., (2011). Structural Network Topology Revealed by White Matter Tractography in Cannabis Users: A Graph Theoretical Analysis, Brain Connectivity. 1:473-83.
Beeri, M.S., Lee, H., Cheng, H., Wollman, D., Silverman, J.M., Prohovnik, I. (2011). Memory activation in healthy nonagenarians. Neurobiology of Aging, 32:515-523.
Cheng, H. & Li, Y. (2010). Respiratory Noise Correction Using Phase Information. Magnetic Resonance Imaging, 28:574-582.
Cheng, H., Huang, F. (2006). MRI Image Intensity Correction with Extrapolation and Adaptive Smoothing. Magnetic Resonance in Medicine, 55:959-966.
Cheng, H., Zhao, Q., Duensing, G.R., Edelstein, W., Spencer, D., Browne, N., Saylor, C., Limkeman, M., (2006). SMARTPHANTOM – an fMRI Informatics Tool. Magnetic Resonance Imaging, 24:301-313.